
Center for Intelligent Robotics
實驗室名稱:精密系統控制實驗室
研究專長:奈米操控、嵌入式系統、精密伺服
Name of Lab:Precision System Control Lab (PSCL)
Research Interest:Nano-Manipulation, Embedded Systems, Precision Servo
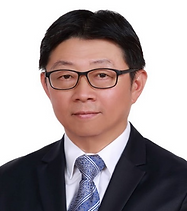
以深度強化學習配合俱模型為基礎控制來達成機器手臂在複雜環境下的任務控制
Task Control of Robotic Arm in Complex Environments Using Deep Reinforcement Learning with Actor-Critic Models
本研究藉由機器學習進行機械手臂軌跡控制,將重複的動作做更精密控制,同時使用不同的方法去做學習,藉此找出最佳的控制器,並比較在機械手臂末端點與姿態誤差,來達成所設定的任務要求。
In this research, machine learning is employed for the trajectory control of a robotic arm to achieve more precise control of repetitive motions. Various learning methods are utilized to identify the optimal controller, and a comparison is made based on the end-point position and pose errors of the robotic arm to fulfill the specified task requirements.
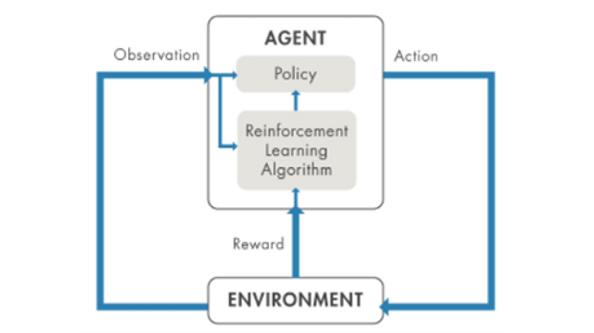
新穎三焦人工水晶體設計
Novel Trifocal Artificial Crystalline Lens Design
本研究設計符合生物相容性的光學透明材料製成之人工水晶體,配合材料以及光學幾何設計製造模具,並藉由台大之單點鑽石切削設備來製作,完成人工水晶體製程,並建立模擬和實驗環境,建立模擬分析比較後,優化製造技術。
Biocompatible Optical Transparent Material for Artificial Crystalline Lens Design: Mold Fabrication, Diamond Cutting Process, Simulation, and Experimental Optimization.

肌耐力增強型動力外骨骼系統研製與驗證計畫
Development and Validation of Muscle Endurance-Enhanced Powered Exoskeleton System
隨著人機互動之應用越來越廣泛,人類意圖偵測也變得漸趨重要。本研究使用機器學習系統進行一般人進行不同動作之意圖預測,其中受試者會在右腿上穿慣性測量單元以量測與計算其各個關節之角度、角速度與角加速度。本研究之結果取得了不錯的成效,我們也調整其它參數等,以及使用同樣的訓練者資料來預測不同受試者的動作意圖,結果顯示即使訓練資料與測試資料來自不同的人,本系統仍然可以進行意圖預測。
With the increasing application of human-machine interaction, human intent detection has become increasingly important. In this research, a machine learning system is used for the intent prediction of different movements performed by the general public. Participants wear inertial measurement units on their right legs to measure and calculate the angles, angular velocities, and angular accelerations of their joints. The results of this study show promising outcomes. We also adjusted other parameters and used the same training data to predict the intent of different participants, demonstrating that the system can still perform intent prediction even when training and testing data come from different individuals.
